A&S Research Travel Awards
Jennifer Delzeit and Jianfeng Chen have each received an Arts & Sciences Research Travel Award for Spring 2019. They will attend the 2019 Eastern North American Region (ENAR) spring meeting (March 24-27, 2019) in Philadelphia, which is a large-scale professional biostatistics conference for the region of Eastern America and expected to attract more than 1000 participants to attend this conference (https://enar.org/meetings/spring2019/index.cfm).
Ms. Delzeit will present her master's research and Mr. Chen will give an oral presentation of his PhD research. Their major professor is Dr. Wei-Wen Hsu. The research is in collaboration with Dr. David Todem from Michigan State University and Dr. KyungMann Kim from the University of Wisconsin-Madison.
Ms. Delzeit has received a job offer and will graduate this May. Mr. Chen expects to graduate in Fall 2019.
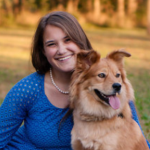
Univariate gradient statistic for marginal cure rate model with high-dimensional covariates
Authors:
Jennifer Delzeit, Jianfeng Chen, Wei-Wen Hsu, David Todem, KyungMann Kim
Abstract:
Cure rate models have been well established and widely used in the literature for analyzing the lifetime data of long-term survivors. Owing to the advancement of genomic technology, it is now of interest to identify the significant genes or microarrays that are highly associated with the survival outcome under the cure rate model framework. The identification procedure using these genetic data will involve the technique of variable selection for high-dimensional covariates. However, the cure rate model requires the additional modeling of the cure fraction, which inevitably leads to a more complicated variable selection process. In this paper, we propose a gradient-statistic-based variable selection method under the marginal representation of cure rate model. This marginal model can produce interpretable covariate effects on the overall survival response by relating the marginal mean hazard rate to high-dimensional covariates directly without specifying the cure fraction component. A univariate gradient statistic is then used iteratively to determine significant covariates. Coupled with the use of a False Discovery Rate approach, the top-ranked list of covariates can be easily obtained. The results of the proposed method are illustrated by extensive simulations and an application to TCGA breast cancer data which contain more than 400,000 microarrays.
Marginal cure rate models for long-term survivors
Authors:
Jianfeng Chen, Wei-Wen Hsu, David Todem, KyungMann Kim
Abstract:
Two-component mixture models for long-term survivors, known as standard cure rate models, have been widely used and intensively discussed in the literature. Much of the attention has been put on to understand the covariate effects on both the cure fraction and hazard rate components of this model. However, it is extremely challenging to interpret the covariate effects on the overall survival response when these covariates are shared by the cure fraction and hazard rate simultaneously. In this paper, we propose a marginal cure rate model that can provide a general framework to investigate the covariate effects on the survival outcome of the whole population. Technically, a novel transformation that can relate the covariates directly to the marginal mean hazard rate is adopted and embedded in the likelihood function of a standard cure rate model. The proposed marginal model is evaluated through extensive simulation studies and illustrated with an application to the liver cancer data from the SEER registry.